MIT Research Bridges Gap Between Pattern Recognition Systems In Humans & Computers
As humans, we are naturally gifted to identify patterns when we are shown only a handful of examples. On the contrary, computer systems require huge data sets to be able to identify pattern. In an attempt to build a bridge in the way information is processed by computers and humans, a team of researchers at MIT have developed a new system that can help computers make better decisions using examples. The system is able to learn from data broken down into examples and drawing judgments out of them. To better understand their idea, take an example of what any man would do when presented with a completely new scenario is a field operation. The man won't start searching possible use-cases like a machine, but come up with a decision bases on previous experiences.
Massachusetts Institute of Technology (MIT) carried out experiments on a supervised machine, which learn from data provided to it and tries to find correlations in it. For instance, they had data with images that had the most occurrences of the parts of a car. The machine could gauge that the data is related in some way, but how it is related is now obvious. In an unsupervised learning system, the person or machine generally relies on previous information known. For example, if presented with a pile of documents, it can tell that the ones that have words such as âtonalityâ and âharmonyâ are related to music theory, while those full of words like âjurisprudenceâ and âappellateâ are legal documents.
The MIT researchers made two major modifications to the type of algorithm commonly used in unsupervised learning. Firstly, they clustered not only on the basis of data itemsâ shared features, but also on their similarity to some representative example called "prototype". Secondly, instead of ranking shared features according to importance, the new algorithm tries to bring the list of features down to a representative set, which the researchers called a âsubspace.â To describe a good subspace, you need to pick up a good prototype and vice versa to make the algorithm work.
The team also tried their hand at a set of experiments to decide if prototype-based machine learning could be used to improve human decision-making. Their attempt was to make the prototype-constrained algorithm better understand the category of the recipe on the basis of a list of ingredients. Their results showed that it performed way better than the conventionally used topic-modelling algorithm.
Source: #-Link-Snipped-#
Massachusetts Institute of Technology (MIT) carried out experiments on a supervised machine, which learn from data provided to it and tries to find correlations in it. For instance, they had data with images that had the most occurrences of the parts of a car. The machine could gauge that the data is related in some way, but how it is related is now obvious. In an unsupervised learning system, the person or machine generally relies on previous information known. For example, if presented with a pile of documents, it can tell that the ones that have words such as âtonalityâ and âharmonyâ are related to music theory, while those full of words like âjurisprudenceâ and âappellateâ are legal documents.
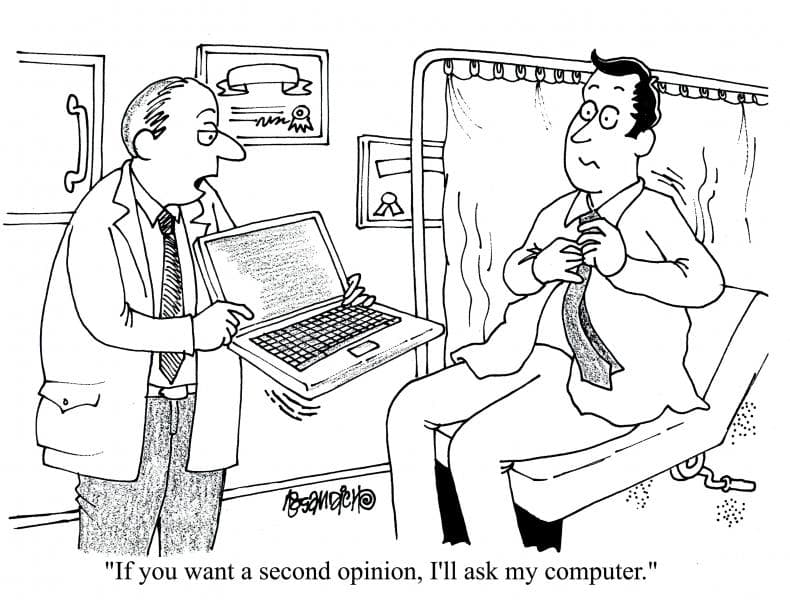
The MIT researchers made two major modifications to the type of algorithm commonly used in unsupervised learning. Firstly, they clustered not only on the basis of data itemsâ shared features, but also on their similarity to some representative example called "prototype". Secondly, instead of ranking shared features according to importance, the new algorithm tries to bring the list of features down to a representative set, which the researchers called a âsubspace.â To describe a good subspace, you need to pick up a good prototype and vice versa to make the algorithm work.
The team also tried their hand at a set of experiments to decide if prototype-based machine learning could be used to improve human decision-making. Their attempt was to make the prototype-constrained algorithm better understand the category of the recipe on the basis of a list of ingredients. Their results showed that it performed way better than the conventionally used topic-modelling algorithm.
Source: #-Link-Snipped-#
0